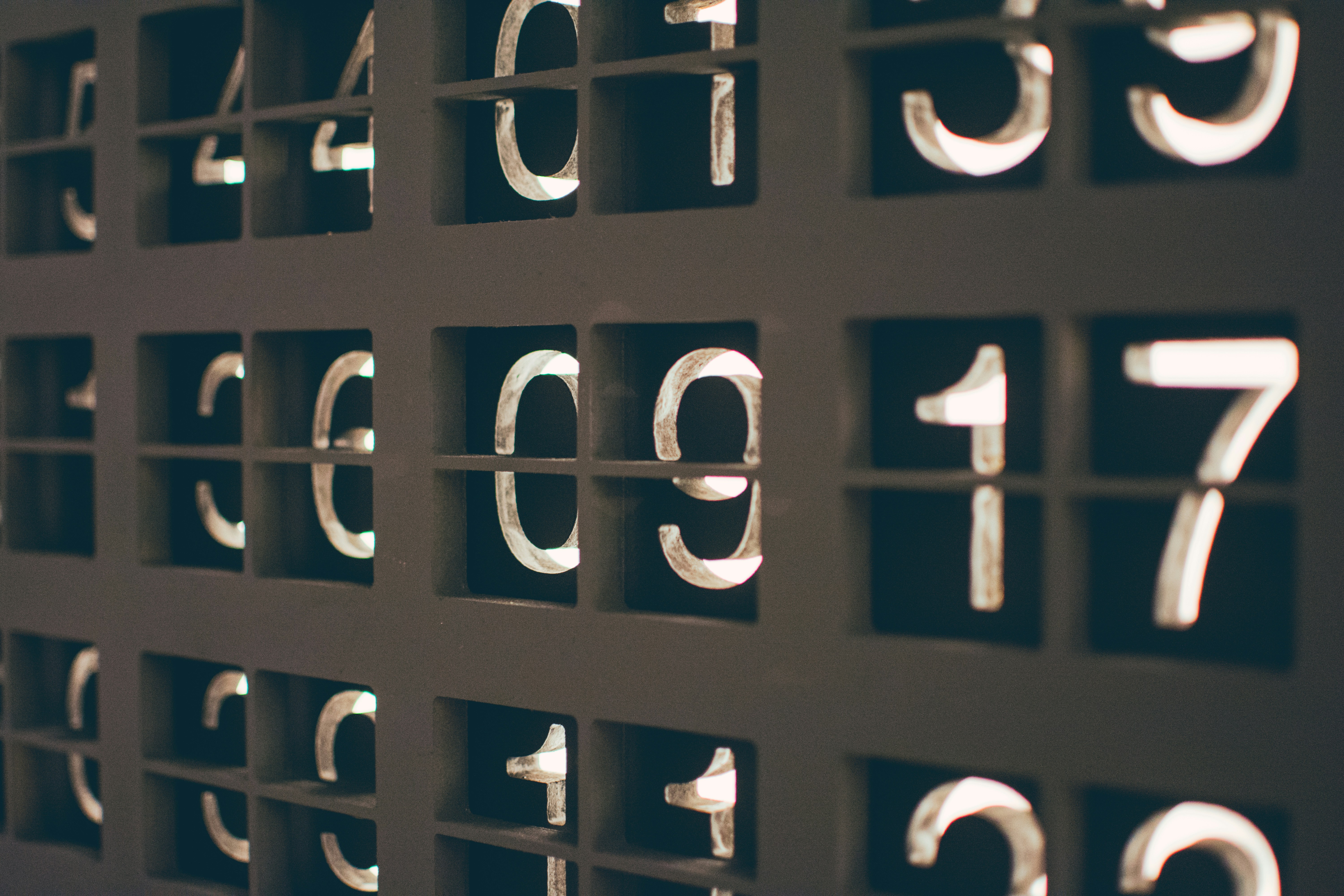
Introduction
In the realm of artificial intelligence and machine learning, vector databases have emerged as pivotal tools, enabling efficient storage and retrieval of high-dimensional data. These databases are particularly adept at similarity searches, which are crucial for applications ranging from recommendation systems to natural language processing. Among the myriad vector databases available, two names stand out: Pinecone and Chroma. Both have carved a niche for themselves, offering distinct features and capabilities. This article aims to delve deep into the intricacies of these two databases, comparing and contrasting their features, to provide a comprehensive understanding for those looking to make an informed choice.
Nature (Open-source vs. Managed)
The fundamental difference between Pinecone and Chroma lies in their nature. Pinecone is a managed vector database, meaning it offers a streamlined experience where much of the backend infrastructure is handled by the service provider. This can be particularly advantageous for businesses and developers who wish to focus on application development without the intricacies of backend management.
Chroma, on the other hand, is open-source. This provides users with the flexibility to modify, extend, and tailor the database to their specific needs. Open-source solutions like Chroma often foster a community-driven approach, where developers from around the world contribute to its improvement, ensuring its adaptability and growth in line with evolving requirements.
Real-time Search Capabilities
One of the most sought-after features in vector databases is the ability to perform real-time searches, especially when dealing with vast amounts of high-dimensional data. Pinecone excels in this domain with its blazing-fast search capabilities. It allows users to retrieve similar vectors in real-time, making it an ideal choice for applications that demand instantaneous results, such as recommendation engines and content-based searching.
While Chroma is efficient, it might not match Pinecone’s performance in certain high-throughput scenarios. However, it compensates with its flexible querying capabilities, which can cater to a broader range of applications, including complex range searches and combinations of vector attributes.
Scalability
For applications anticipating growing data and traffic demands, scalability becomes a paramount concern when selecting a vector database. Pinecone’s architecture is meticulously designed with scalability in mind. It can effortlessly scale with increasing data and traffic, making it a prime choice for high-throughput applications that grapple with vast data volumes.
Chroma, being open-source, offers scalability but might require more hands-on management and expertise to ensure optimal performance at scale. While it can handle growth, the onus is more on the user or the organization to manage and optimize the infrastructure, especially when compared to a managed solution like Pinecone.
Indexing Mechanism
The efficiency of a vector database is often determined by its indexing mechanism, which plays a pivotal role in storing and retrieving data. Pinecone stands out with its automatic indexing feature. This reduces the burden on developers by automating a crucial step, thereby simplifying the deployment process. The automatic indexing ensures that vectors are organized optimally, facilitating faster retrieval.
Conversely, Chroma’s open-source nature offers more flexibility in its indexing approach. Users have the liberty to customize the indexing process based on their specific needs. However, this might also mean a steeper learning curve and the need for more hands-on management to ensure optimal indexing.
Querying Functionality
Like all databases, querying functionality is central to vector databases. The ability to execute complex queries efficiently can significantly impact the user experience and the overall utility of the database.
Chroma excels in this domain with its extensible querying capabilities. It allows for more intricate querying, including complex range searches and combinations of vector attributes. This flexibility makes Chroma suitable for a broader spectrum of applications, catering to nuanced requirements that might go beyond simple similarity searches.
Pinecone, while exceptional at similarity search, might have some limitations when it comes to advanced querying capabilities. Its primary strength lies in retrieving similar vectors swiftly, but for projects that demand more intricate querying functionalities, Pinecone might not be the first choice.
Support and Integration
Integration capabilities and support can significantly influence the adoption and usability of a vector database, especially in diverse tech ecosystems.
Pinecone offers a seamless experience, especially for those familiar with the Python ecosystem. Its easy-to-use Python SDK ensures that integrating Pinecone into applications is straightforward. This support is invaluable for those looking to quickly deploy and iterate on their applications without steep learning curves.
Chroma, being open-source, benefits from a vibrant community of developers. This community-driven approach means that Chroma often has a plethora of integrations and support tools developed by its users. While it might not have a dedicated SDK like Pinecone, the community often steps in to provide SDKs, plugins, and other tools that facilitate integration into various platforms.
Cost and Pricing Structure
For many organizations, especially those considering long-term commitments, the financial aspect of adopting a technology solution is often decisive.
Pinecone, being a managed service, operates on a pricing structure that might vary based on usage, data volumes, and other factors. While it offers the convenience of a managed solution, the costs can become a concern, especially for large-scale deployments or startups with tight budgets.
Chroma, on the other hand, being open-source, doesn’t come with licensing fees. However, it’s essential to consider the indirect costs associated with it. Setting up, managing, and scaling an open-source solution like Chroma might require dedicated resources, both in terms of manpower and infrastructure. While there might not be direct costs associated with the software, the total cost of ownership could include expenses related to customization, maintenance, and potential scaling challenges.
Conclusion
Vector databases, with their unique capabilities, have become indispensable tools in the AI-driven landscape. Both Pinecone and Chroma offer robust solutions for storing and retrieving high-dimensional data, each with its distinct features and strengths. Pinecone, with its managed service approach, provides ease of use and real-time search capabilities, making it a go-to for businesses seeking immediate deployment without backend hassles. Chroma, with its open-source nature, offers flexibility and a community-driven ecosystem, catering to those who prioritize customization and hands-on management. Choosing between them boils down to specific needs, budget considerations, and long-term goals. Both databases, however, stand as testament to the advancements in the realm of vector data storage and retrieval.